Quick-Start Synopsis – MLR is a bottleneck and technology can help
Leveraged correctly, technology like AI and machine learning should enable us to do mundane tasks quicker. One of the tasks that internal teams in Medical Affairs spend a lot of time and effort around is Medical–Legal-Regulatory (MLR) review – or however your team refers to it internally. If teams are not using MLR for scientific content, they certainly review marketing and promotional pieces through this pathway.
Machine learning (ML) and artificial intelligence (AI) are now being leveraged within Medical Affairs teams to shorten the time dedicated to this process.
Have you considered doing the same for your team? A recent webinar from MAPS discussed their experience in a pilot study leveraging AI.
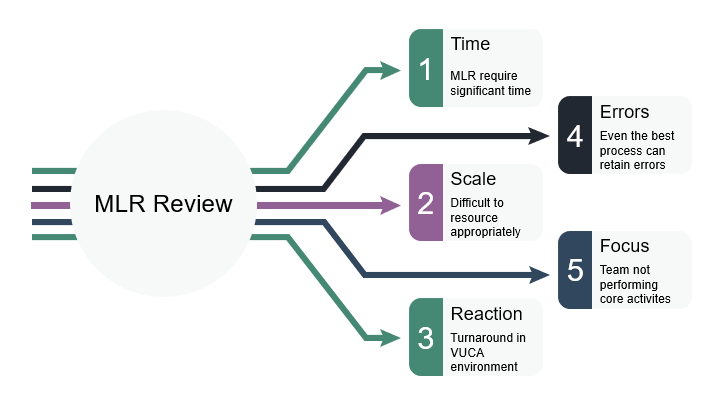
What is artificial intelligence?
Artificial intelligence sounds like technology from a Sci-Fi movie, but it is already all around us. You likely engage with artificial intelligence daily, even if you are not aware of it. Any computer software that performs “human-like” activities is in this category.
- Customer Relationship Management (CRM) systems use AI to self-update over time – decreasing time to maintain the database.
- Do you use facial recognition to open your phone? Then you are using AI.
- What about those algorithms that define your social media feeds? AI drives these tools. You see more of what you liked and the computer software recognized and adapted based on that information. Of course, my mom still thinks all feeds are the same!
- Spam filters at work for email? They operate with AI as well as anti-virus software.
- Google searches search the entire Internet for you and provides results based on artificial intelligence.
What is machine learning?
Machine learning is a subset of artificial intelligence. Machine learning helps computers process vast amounts of data quickly. The software allows the system to get better at processing over time. Machine learning is useful as more and more data becomes available and stored in data lakes across enterprise organizations and in the cloud.
MLR requires a lot of team bandwidth
Want to learn more? Here’s a deeper dive. Medical-Legal-Regulatory review can take months for a single piece of content. Multiple stakeholders review and validate information, data, and claims/messaging for each asset. Despite the number of eyes on a single piece, MLR-approved files may still have errors. One pharma company found that approximately 25% of MLR-approved files had errors introduced during the review process.
Is there a better, faster, and more efficient way to process items through MLR? Doing so would remove a bottleneck within an organization’s Medical Affairs.
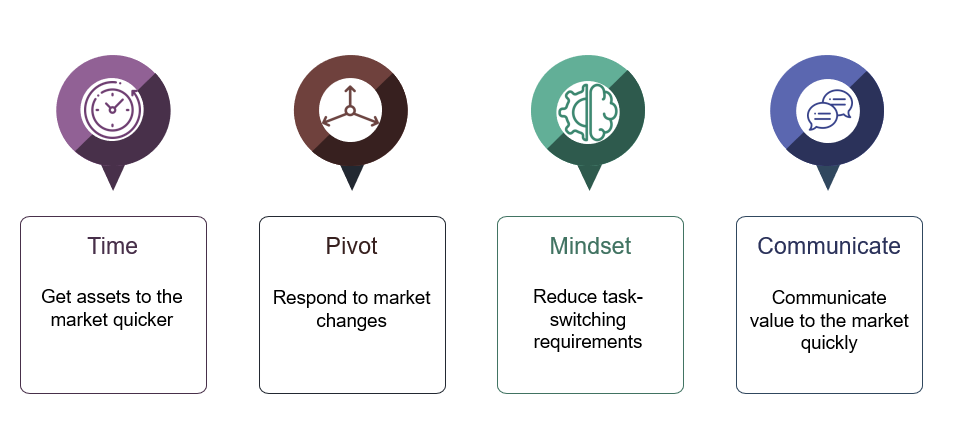
The current review process can result in several negative business outcomes:
- A long time to get assets to market
- Inability to pivot due to market changes when needed
- Difficulty switching between a promotional review mindset and a medical review mindset when members serve in both capacities
- Delay in communicating value to the marketplace
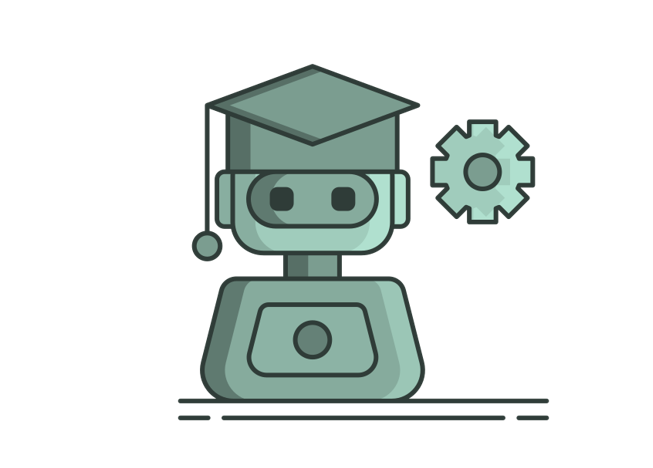
Providing additional resources as a short-term solution
Some companies offer additional human resources to Medical-Legal-Regulatory review – particularly when many assets require review before a launch or a large shift in the data story. For example, providing a high-performing MSL a stretch assignment as part of the MLR team. However, that often falls short.
Individuals brought into the team for a short-term stint may not be trained to review pieces, especially when it comes to objective, factual statements versus subjective claims.
Likewise, medical editing is a skill that may not be developed sufficiently in the internal team to enable an individual contributor to take on this additional role.
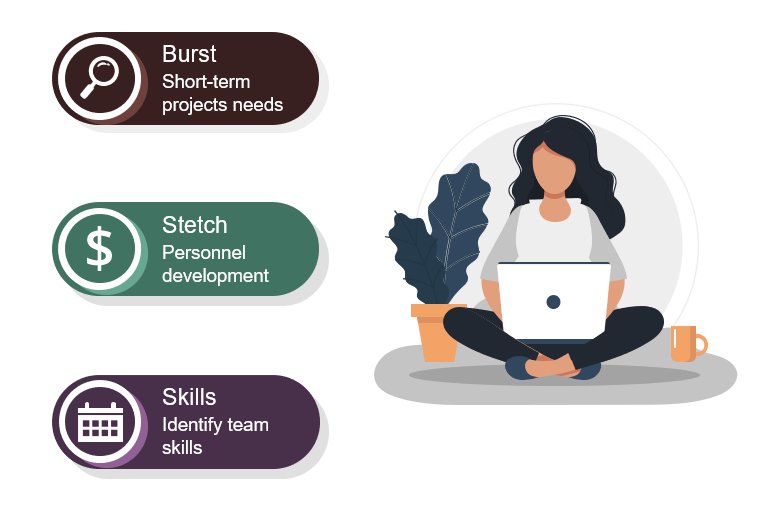
Off-load MLR review to an external resource
Other companies shift the burden of MLR to an external vendor for some or all of the load. The scope includes having the vendor annotate and anchor supporting references in Veeva (or similar platforms), create a database of claim statements, and perform the medical editing and data checks.
This option may be effective for companies that do not have many assets moving through MLR or have the budget available to outsource the process. Still, outsourcing is not a viable option for many pharmaceutical companies.
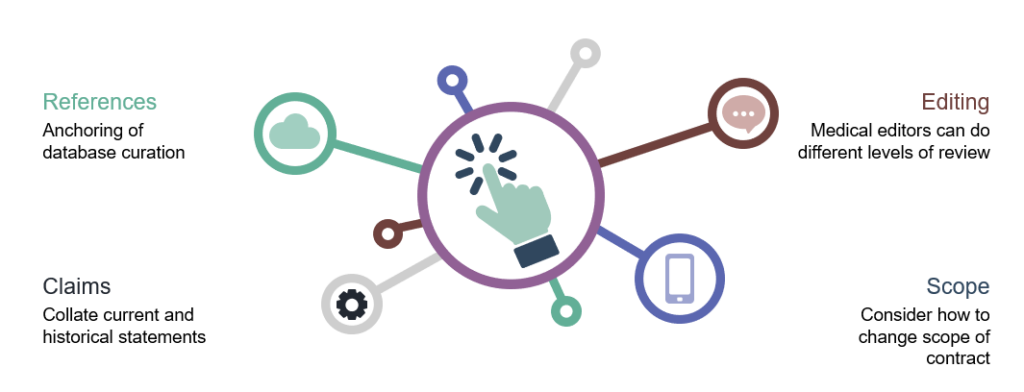
Artificial intelligence and machine learning platforms can help medical affairs
What has become a viable option recently is to leverage AI platforms. It is now possible to create a claims management database with all associated references associated with the claim linked directly in the platform.
When the platform reviews the asset, claims not fully supported by referenced materials are highlighted for review.
The MLR team can then determine whether the statement needs to be changed or provide reference material that supports that claim. The system then adds the new reference to the database so that it is available for future assets. The platform demonstrated can identify statements that are partially supported.
Solutions differ among vendors – Watson Health AI, GE Healthcare, Monocl, Indegene, Nexocode, IQV IA, Sorcero, Innoplexus, Anju, and others. The list is growing all the time.
The technology provides consistency in how assets are reviewed and decreases time requirements and workload for the MLR team.
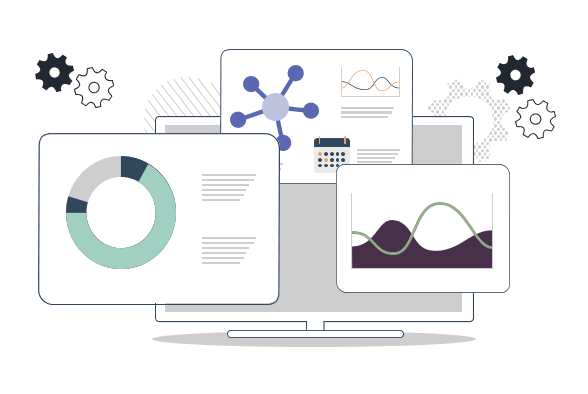
Quickly Compare Versions of a Document Down to the Decimal Point
A related function of AI platforms is that they can compare content between assets. Image recognition has improved dramatically – consider all the apps that now identify your houseplant or a bird in your backyard.
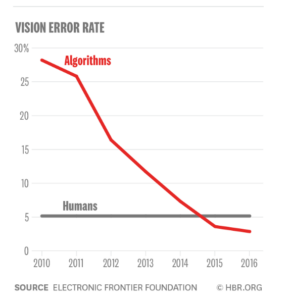
In MLR review, AI platforms can:
- Recognize differences between an asset created at the beginning of a review process and the asset after several rounds of review
- Streamline curation efforts when replacing one asset with completely new content. Why map each change when the system can do it for you?
The system identifies any mismatches between the two versions. It is similar to the “view side-by-side” option in Windows but in an advanced and more dynamic application.
In the example demo I viewed, the system picked out:
- Text changes
- Graphic modifications (the addition of another patient avatar within a composite graphic)
- Significant figure differences
- New text and content
- Differences in the references used to support statements
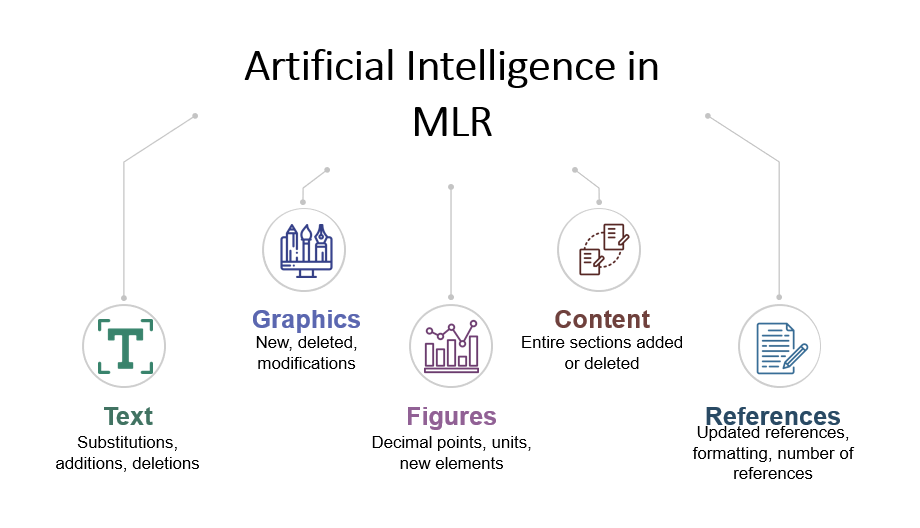
Training the platform requires time, patience, and materials
Setting up the platform takes work and requires that the platform “learn” from the added materials. That is how machine learning differs from the traditional “coding” of computer software.
On a positive note, the number of items necessary to train the platform using content from the Medical Affairs team was much smaller in scope than the number of assets required for marketing or sales pieces. Training requirements are key because some large systems in the past required millions of correctly scored examples. I hope that no MLR team has millions of previously reviewed assets lying around!
The beauty of machine learning is that the system can perform tasks on its own. Innovative teams will capitalize on this general purpose technology and extend their team’s capabilities and expertise. AI technology is waiting to be scaled and used across a range of processes in our work lives.
It will be very interesting to see this technology develop over time and observe how Medical Affairs departments can gain value by leveraging AI and machine learning as part of their operational processes.
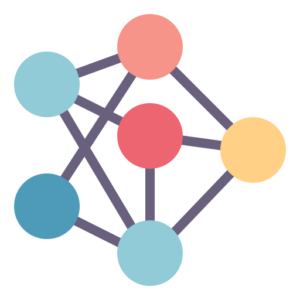
Proven Value for Medical Affairs
Medical Affairs continues to grow in importance and value within organizations.
As new responsibilities accrue, the team will need to carefully consider mechanisms that improve operations and allow team members to provide internal value while maintaining focus on high-value responsibilities such as KOL relationship-building and insight generation.
Medical’s desire to focus on strategic tasks that benefit the company’s strategic imperatives should leverage technology platforms including ai and machine learning for medical affairs.
Contact Us to Develop Your Strategy
Versus Learning Solutions focuses on supporting your Medical Affairs team. Our primary goal is to provide high-quality, custom training for your team.
We can assist you with deciphering the many options that are available in the AI marketplace.
References
Medical Affairs Professional Society. Learnings from a practical experience of using NLP/AI in Medical Affairs. Digital Strategy FAWG webinar. April 28, 2021.
Brynjolfsson, Erik and Andrew McAfee. How AI Fits into Your Science Team. Harvard Business Review. 2017 July. https://starlab-alliance.com/wp-content/uploads/2017/09/The-Business-of-Artificial-Intelligence.pdf